

17- 20 As the prevalence of large multidimensional datasets continues to grow, the ability to manually take measurements not only becomes impractically time-consuming, but the sensitivity, accuracy, objectivity, and reproducibility of doing so can become greatly inhibited. 15, 16 Image analysis also serves a crucial biomedical role for diagnostic interpretation.
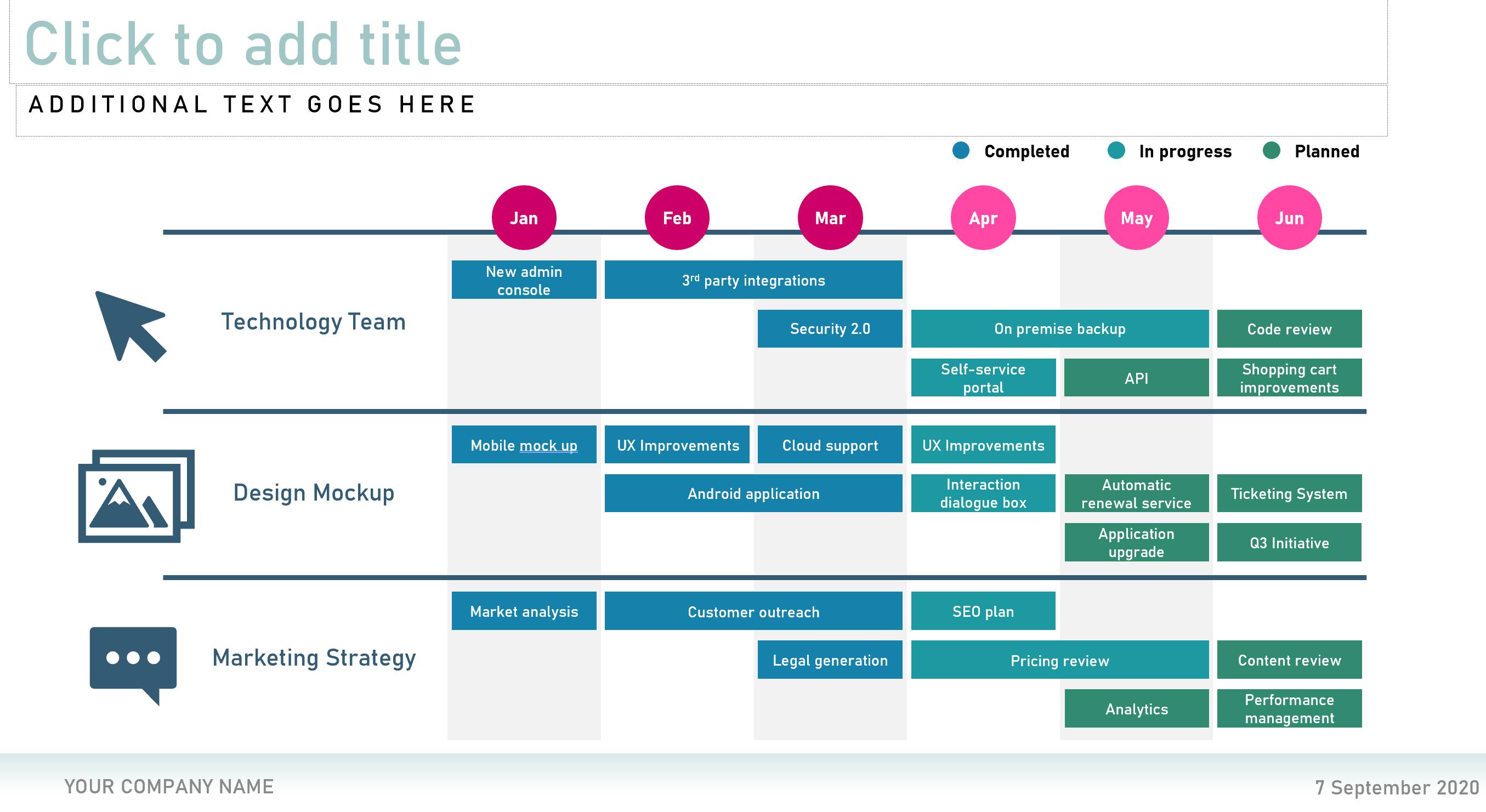
8 This is important for a wide array of biological studies that include quantifying the proximity of fluorescent-labeled proteins, 9 tracking cell fates over time, 10 automating cell counting, 11 tracking invading cancer cells, 12 collecting whole-slide information, 13 quantifying and characterizing cells, such as microglia, within the brain, 14 or registering multiview light sheet fluorescence microscopy datasets to study development. 3- 7 Modern research therefore requires methods for the efficient and robust manipulation, interpretation, and visualization of such advanced, multidimensional imaging data. Novel imaging modalities offer enhanced resolution, specificity, and coverage and have contributed to many of the tremendous biological advancements over the past several decades. 2 To address this need, open source software is advantageous, and this is one of the main reasons for ImageJ's widespread use.
#Download free software modicon concept 26 software full#
If any component of the algorithm is proprietary, researchers may be unable to reproduce their work or track changes with full transparency, which is imperative to the scientific process. For this to occur, the algorithms and parameters used must remain open and consistent. 2 Image analysis allows users to extract information from images in a reproducible manner. 2 As novel imaging modalities emerge and datasets become more complex, having reproducible and reliable methods to interpret biological images is the core of image analysis, where “seeing is a matter of learning”. 1 Imaging-based methods serve a crucial role in the life sciences and have undergone tremendous growth in the past decades. ImageJ is a widely-used open-source software that allows users to visualize, inspect, quantify, and validate scientific image data. Therefore, we also discuss the contributions of ImageJ2 to enhancing multidimensional image processing and interoperability in the ImageJ ecosystem. These new tools have been facilitated by profound architectural changes to the ImageJ core brought about by the ImageJ2 project. Moreover, new capabilities for deep learning are being added to ImageJ, reflecting a shift in the bioimage analysis community towards exploiting artificial intelligence. These plugins and tools have been developed to address user needs in several areas such as visualization, segmentation, and tracking of biological entities in large, complex datasets. Here we review the core features of this ecosystem and highlight how ImageJ has responded to imaging technology advancements with new plugins and tools in recent years. We refer to this entire ImageJ codebase and community as the ImageJ ecosystem. It is available in many forms, including the widely used Fiji distribution. ImageJ consists of many components, some relevant primarily for developers and a vast collection of user-centric plugins. The close collaboration between programmers and users has resulted in adaptations to accommodate new challenges in image analysis that address the needs of ImageJ's diverse user base. ImageJ is an open-source image analysis software platform that has aided researchers with a variety of image analysis applications, driven mainly by engaged and collaborative user and developer communities. As techniques for acquiring images increase in complexity, resulting in larger multidimensional datasets, imaging software must adapt. For decades, biologists have relied on software to visualize and interpret imaging data.
